Dev Diary 11: Demo day and a response to Archaeological HARKing
FAIMS 3 participated in the MQ Incubator's Demo Day! Brian also takes an opportunity to respond to a blog post about HARKing, and share a few dev updates!
In this dev update, we share a brief update about the MQ Incubator’s Demo Day and reflect on Jeremy Huggett’s recent blog on: HARKing to Big Data?
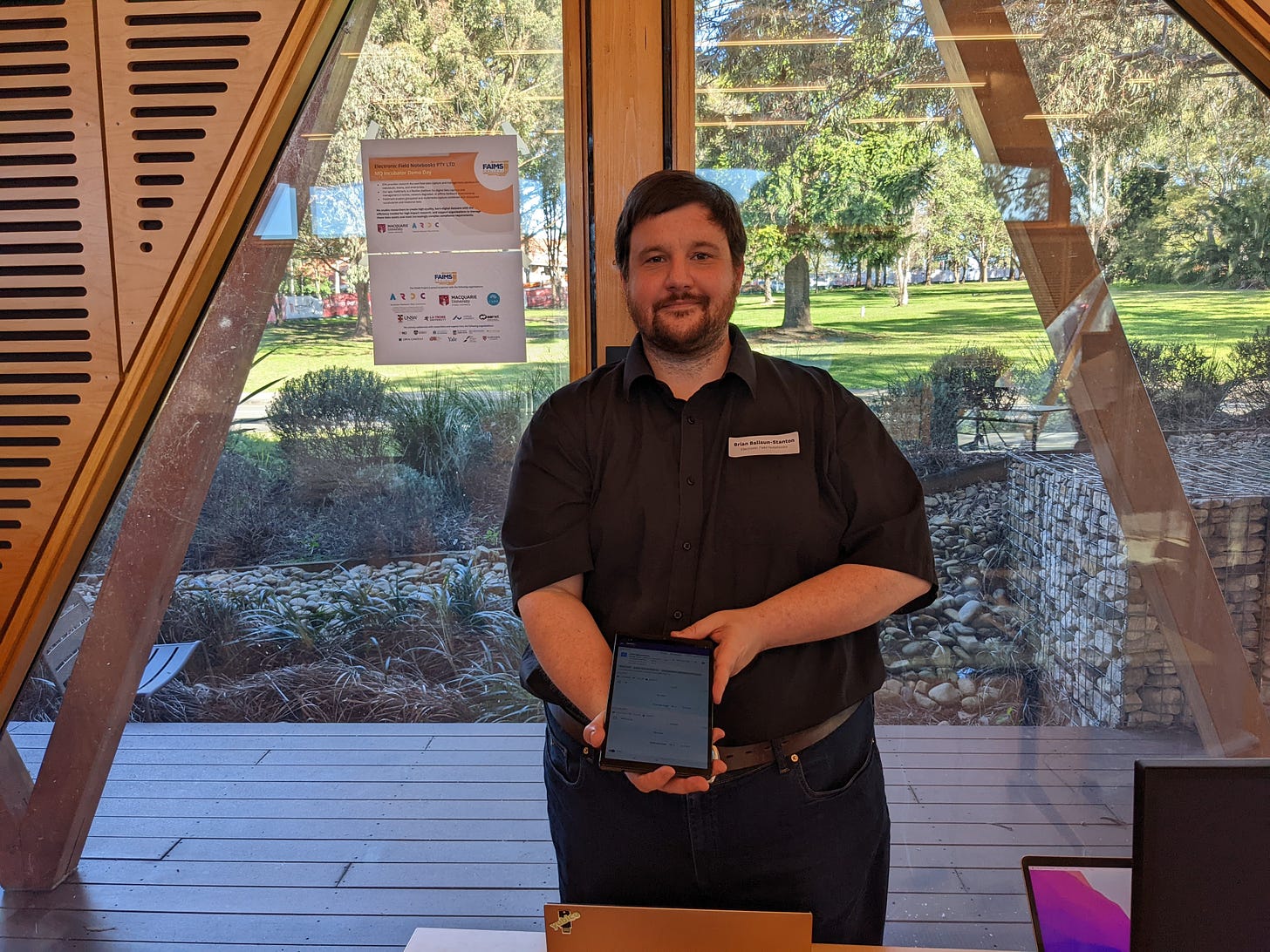
Status update
Planning continues for our November production release. In the last two weeks, we’ve mostly been cleaning up and releasing to production for PSMIP Data. We’ve also started work on redoing our authentication services to make sure we can hook into multiple OAuth providers.
You can see our latest work on Google Play, so long as you’ve registered with DataCentral and signed up on our demo registration form so we can give you access!
In something that makes me personally quite pleased, one of our collaborators at Macquarie University (Tess Nelson) independently developed a second Groundwater Pump Testing notebook on her own initiative, without needing support from me! We have already met FAIMS2’s record for independently created field notebooks!1
Demo Day
The Electronic Field Notebooks team also attended the MQ Incubator demo day last Thursday! It was mostly a chance to practice our short pitches and realise that our traditional hour-long demo presentation … doesn’t map to running a corner of a table and trying to get folks wandering around interested in our product. Many lessons still to be learned there.
For online demos, we’re thinking of making 5-minute videos around various features of FAIMS 3. What do you folks think about that length. Too long? Too short? What would you want to hear in a pitch for electronic field notebooks?
A response to ‘HARKing to Big Data’
First, to “HARK” is to “Hypothesise after the results are known” (As discussed in our book chapter discussed in Dev diary 6):
Here, I have a few thoughts in response to Jeremy Huggett’s HARKing to Big Data:
None of these [hypothesis generating practices] sound like good practice, especially the idea of deliberately (and quietly) setting hypotheses aside that don’t work out. But it isn’t quite so simple. Forms of HARKing are quite common in archaeological practice. We’re familiar with searching for patterns within our data, deciding which ones seem to be useful, and then hypothesising about what they might mean about past lives and activities. It is, after all, related to the process of scientific induction, where conclusions are drawn from patterns observed in the data, although this is not without its problems (see Smith 2015, 19, for example, and the discussion in Ross and Ballsun-Stanton 2022).
In our discussion in Digital Heritage and Archaeology in Practice, we say2 (page 3):
Indeed, “using current results to construct post hoc hypotheses that are then reported as if they were a priori hypotheses”, “failing to report a priori hypotheses that are unsupported by the current results” (Rubin 2017), or “presenting exploratory work as though it was confirmatory hypothesis testing” (Fraser et al. 2018), is considered “hypothesizing after the results are known” or HARKing (Kerr 1998). HARKing is always a questionable research practice if it is unreported, although disagreement exists about the acceptability (or even desirability) of careful and transparent post hoc analysis in deductive research (Rubin 2017; Hollenbeck and Wright 2017). In a 2018 paper surveying over eight hundred ecologists and evolutionary biologists, 51% admitted to HARKing (Fraser et al. 2018). Fraser also notes in passing that when published papers fail to disclose a priori hypotheses (or if there were a priori hypotheses), it becomes difficult to judge whether HARKing – or the conflation of postdiction and prediction more broadly – has even taken place. As such, the a priori articulation of hypotheses required by preregistration (or the explicit statement that research is inductive) can help to combat this species of questionable research practice.
A brief recap of research modes:
Hypothesis-testing research, deductive research, is taking a known general case and deriving conclusions from it. Deductive reasoning is tautological. Deductive, predictive approaches in research are useful — as testing one’s assumptions and if the general case holds in a particular instance is one way of falsifying a risky statement3.
Hypothesis-generating research, inductive research, is reasoning from specific instances to a general covering case. It is the practice of keen observation and observing trends in nature. Case studies are a great example of this sort of research in a qualitative context.
Abductive research engages in rapid hysteresis between inductive and deductive research, exploring and narrowing down a possibility space for future investigation. Pragmatically, I expect some research projects utilise abductive methods either during initial conception or during writeup when scrambling for a literature. I have seldom seen the practice used explicitly and with prior intent.
Huggett explores around the concept of Corrigibility when he says:
So there’s no problem with big data mining and allied artificial intelligence related methods of analysis which hypothesise after the results, as long as we are transparent about this, then? Not entirely. If the algorithms used are black-boxed, or the internal procedures so complex as to defy understanding, and the systems incapable of explaining their reasoning (e.g., Huggett 2021, 424-428) then the transparency required of ‘good’ HARKing cannot be achieved. There is, therefore, no substitute for human intervention in the process of analysis, to understand and to evaluate the outcomes, and to determine whether the patterns and relationships identified are actually valid as well as useful. (Emphasis mine)
A corrigable “AI” (or ML system) is one that is inspectable and interrogatable as to its methods. Certainly, the current practice in ML training is to reserve a portion of the training dataset for verification to ensure that the system is being trained appropriately. Moving away from AI, to “Big Data4” this sort of correlational investigation fits poorly into the inductive mould. Documenting correlations in “large” datasets may be useful, if not real. Huggett then discusses the need for ground truthing:
Of course, human intervention is a feature of many current archaeological applications of neural networks, data mining etc., typically evidenced in a concern for ground truthing and correcting the algorithms and the patterns that the systems latch onto. But this concern is often seen in terms of training the system, implying that the responsibility for the outcomes will ultimately be transferred to the system and in the process setting aside the role of the human expert in validating the patterns and the conclusions that may be drawn from them.
In one sense, we can consider this an abductive process: the inferences produced by machine are validated “in the real”. Having just supported a team going on a survey, I see some similarities with the normal field experience of folks with FAIMS modules/notebooks: there tend to always be tweaks required when the notebook hits the field that were not previously anticipated in the research design.
From my reading of this essay, I see a few themes:
Using algorithmic black boxes to conflate correlation with causal induction
Unreported archaeological “tweaking” of protocols while on the dig site as a form of HARKing and the Gattigla discussion of setting aside hypotheses while observing
The danger of using “big data” claims as an invisible HARKing practice that is proof against corrigibility/transparency while maintaining the pretence of hypothesis testing activities.
First: I believe that sufficiently advanced applied statistics (i.e. ML) can indeed find causal links amongst correlational links. Specifically, that while every correlation is not a causation, every causation has correlated interactions. (By virtue of being a cause, it causes a change in a dependent thing which could be described as a correlated pattern.) However, from my perspective of making field notebooks—Archaeological data is entirely a small data domain. The current means of investigation for survey, excavation, experimental do not admit to the kind of automation at scale that would create an environment for standardisation of data to the level needed for ML. Not to say that other disciplines use ML techniques (Remote sensing comes to mind, as well as vision techniques in pottery sorting and apps like ArchAIDE) are not useful—they certainly aid in novel knowledge creation, but that the present reward systems create few incentives for consistently encoded knowledge representations of archaeological results.
This is entirely OK. Not everything needs to have a Netflix recommendation engine prize5 as the basis for interaction and knowledge production.
As I write this, it feels like an error is being made (either by me, my reading, or Huggett). Looking at the ML techniques above, they are all tools. Right now, they are tools used by humans towards knowledge production, academic goals, and as an excellent excuse to play in the dirt6. Huggett argues:
Gattiglia essentially proposed that theory is set aside temporarily and comes back to the fore following the data analysis, whereas I suggested theory cannot be set aside – like it or not, whether recognised or not, theory is involved at every stage from the recognition, selection, collection and recording of the data onwards[.]
Drawing on my research in the Philosophy of Data (Ballsun-Stanton 2012, 348)7 the structure of the data store informs the shape of the knowledge. Huggett says that theory is involved in every stage—and there I agree. But creating a data store and letting a ground-up investigation of the data provide the impetus for an abductive investigation of what a site or landscape tells you strikes me as a valid approach?
Specifically, we have not yet achieved Artificial General Intelligence8—which means that claims about system-based knowledge production are not valid claims. While triplestores and other knowledge representations may be capable of deductive reasoning, our tools exist to facilitate our searches. Our methodologies may be as meta as we like: from going to a dig with a noticeably clear idea of its history and well-formed data collection methodology to approaching a landscape with a nice notebook in hand for a good session of Slow Archaeology. The tools we use (a nice leatherbound notebook, ML based vision systems, electronic field notebooks, … even excel) are not our results. Both Ackoff and Tuomi’s hierarchies/ontologies of data (see Ballsun-Stanton 2012 for a longer discussion of ontologies of data, information, and knowledge) admit to a relationship between knowledge and data—be it hierarchical or cyclic. An uncritical use of a tool is never a good idea. But making a space for inductive or abductive research as part of the knowledge production cycle remains a theoretically valid knowledge creation activity. Correlation may still have useful pointers for further investigation, even if archaeology has no risk of producing Big Data.
Fundamentally, I agree with Huggett’s conclusion: we must never forget the human researchers are responsible for their outputs. A machine, a tool, an ML approach is only as effective as its designers and reflects them and their theoretical approaches to knowledge—even if the designers make some sort of claim to objectivity.
Stuff I’m reading
(Youtube) Joseph’s Machines — Pass the Wine
In terms of ML, I got a 6/10 when trying to differentiate Daniel Dennett from a computer
On ritual, friction, and formality when shipping software
I discovered a while ago that all those errors and bugs that only appear when you demo something to an audience also magically appear when you record yourself demoing it to nobody. Maybe narrating a feature to a pretend audience takes the blinders off enough that you notice little mistakes you wouldn't have otherwise.
Karaterobot via Simon Willison
Using fractional measures, we can say “A bit more than 1” for FAIMS2, since all modules required our developer intervention and/or support requests. I’m also not counting in-FAIMS edits and tweaks. Measures are hard.
In a much more formal register than this blog…
No, I’m not a positivist. But Sir Karl does make a handy 2x4 in these sorts of conversations, even though his falsificationism has little to do with modern practice in the lab. See: Latour.
My only working definition of big data: “It doesn’t fit on my laptop”
This is one of the notable correlational triumphs of “Big Data” — it was a contest to make better recommendations from netflix viewing habits.
I will leave the relative weighting of these categories as an exercise to the reader.
It looks like UNSW’s library copy of my dissertation is no longer available… Yay for personal archives!
No, that one ex-googler’s claims are not of an AGI system.
5 minute explainer videos for sure! That's about my attention span if I had to be honest :-)
Explainer videos are a great idea! There's an easy way to make them using PowerPoint would you believe - captures your screen and your voice (although I'm sure there's software for that too!). I'd aim for 3 minutes or shorter if you can.